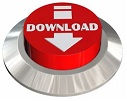
Īutomatic camera-assisted monitoring of insects for abundance estimations is crucial to understand and counteract ongoing insect decline. Source code, data, and models are publicly available at. Lastly, we propose a efficient solution to the over- fitting problem by feeding edge images to the neural networks instead of the images. Furthermore, we introduce a new dataset based on the popular ImageNet dataset as a new bench- mark for comparison. Additionally, we investigate which parts of this process are responsi- ble for overfitting, and apply an explainability tool to further investi- gate the behavior of the trained models. This paper shows that these approaches tend to overfit completely on the synthetic data generation process used to train such networks. Especially in the last few years, solving the radial distortion correction problem from a single image with a deep neural network approach increased in popular- ity. Nonetheless, it is still important to cor- rect that image for radial distortion in these cases. However, some- times it is impossible to identify the type of camera or lens of an image, e.g. It is possible to rectify images accurately when the camera and lens are known or physically available to take additional images with a calibration pattern. Radial distortion correction for a single image is an often overlooked problem in computer vision. Our approach outperforms CAMs on the MS COCO object detection dataset by a relative increase of 27% in mean average precision. Even though the network has only been trained for single-label classification at the image level, DCMs allow for detecting the presence of multiple objects in an image and locating them.

We find that CNNs trained with the cosine loss instead of cross-entropy do not exhibit this limitation and propose a variation of CAMs termed Dense Class Maps (DCMs) that fuse predictions for multiple classes into a coarse semantic segmentation of the scene. This limitation is a consequence of the missing comparability of prediction scores between classes, which results from training with the cross-entropy loss after a softmax activation. However, while CAMs can localize a particular known class in the image quite accurately, they cannot detect and localize instances of multiple different classes in a single image.
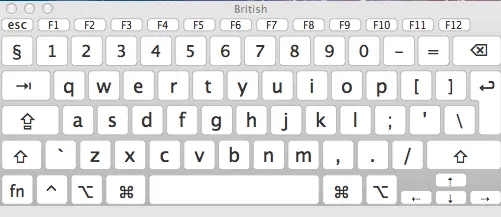
Can we learn to localize objects in images from just image-level class labels? Previous research has shown that this ability can be added to convolutional neural networks (CNNs) trained for image classification post hoc without additional cost or effort using so-called class activation maps (CAMs).
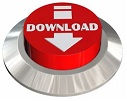